Commuting Behavior at the TU Dortmund 2022
Key data on the survey
From mid-February to mid-March 2023, TU Dortmund University's Sustainability Office conducted a survey on the mobility behavior of members of the university. The aim of the survey was to determine the commuting behavior of university members. To this end, information was collected on the distances traveled during and outside of lecture times, as well as on the preferred means of transport and the time required for this.
A total of 573 (13.7 %) employees (including academic staff) and 897 (2.7 %) students took part in the survey. The total figures for the individual status groups are taken from TU Dortmund University's annual statistical report for 2022. Of the respondents, 48% (58%) of employees (students) are women, 51% (41%) are men and 1% (1%) are diverse.
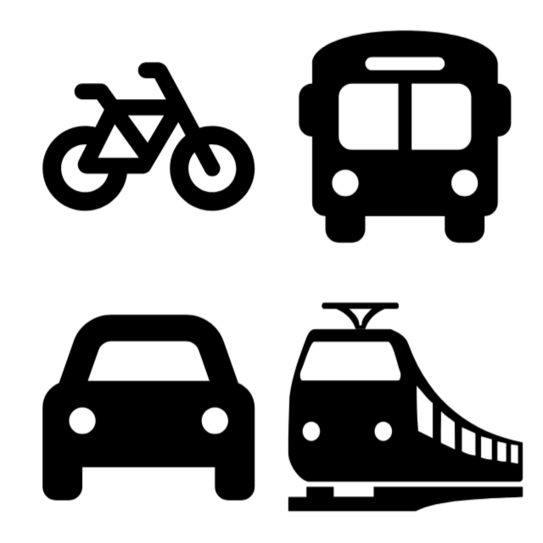
Comments on the Evaluation
The data was analyzed for each question, meaning that the basic populations differed in some cases. Only complete information and plausible answers were analyzed. Due to these quality requirements, in some cases up to 25% of the responses (370 questionnaires) had to be excluded from the analysis. Nevertheless, at least 1000 responses per question could always be taken into account, meaning that the results are still representative.
Commuting Distances, Durations and Frequencies
Interactive graphic. Source: Sustainability Office/TU Dortmund University
A color-coded thematic map of NRW shows the distribution of commuters based on their place of residence. The cities of the federal state are shown. The colors correspond to a logarithmic scale proportional to the number of residences.
- Dark purple: regions with the highest number of residences (logarithmic value around 3).
- Green to yellow: Regions with medium to low residential density.
- Yellow: Regions with the lowest values (close to 0 on the log scale).
The map shows Dortmund as the central metropolitan region, marked by the darker colors, while the surrounding cities have a lower density.
Exact figures are listed in the table below:
City | Number of residences |
---|---|
Dortmund | 802 |
Bochum | 98 |
Essen | 48 |
Witten | 40 |
Hagen | 33 |
Kamen | 18 |
Kamen | 18 |
Hamm | 17 |
Recklinghausen | 17 |
Iserlohn | 16 |
Castrop-Rauxel | 15 |
Lünen | 15 |
Unna | 15 |
Schwerte | 14 |
Gelsenkirchen | 13 |
Duisburg | 10 |
Waltrop | 9 |
Wuppertal | 9 |
Fröndenberg/Ruhr | 8 |
Münster | 8 |
Hattingen | 7 |
Oberhausen | 7 |
Werne | 7 |
Arnsberg | 6 |
Cologne | 6 |
Menden | 6 |
Selm | 6 |
Ennepetal | 5 |
Lüdighausen | 5 |
Soest | 5 |
Werl | 5 |
Weather | 5 |
Dates | 4 |
Dülmen | 4 |
Ense | 4 |
Herdecke | 4 |
Marl | 4 |
Oer-Erkenschwick | 4 |
Sprockhövel | 4 |
Ahlen | 3 |
Altena | 3 |
Bönen | 3 |
Bottrop | 3 |
Gevelsberg | 3 |
Gladbeck | 3 |
Herten | 3 |
Schwelm | 3 |
Steinfurt | 3 |
Ascheberg | 2 |
Bielefeld | 2 |
Gütersloh | 2 |
Halver | 2 |
Holzwickede | 2 |
Moers | 2 |
Remscheid | 2 |
Anröchte | 1 |
Balve | 1 |
Bergkamen | 1 |
Bochholt | 1 |
Bonn | 1 |
Coesfeld | 1 |
Dinslaken | 1 |
Enningerloh | 1 |
Erwitte | 1 |
Grevenbroich | 1 |
Haltern am See | 1 |
Heiden | 1 |
Heiligenhaus | 1 |
Heinsberg | 1 |
Hennef | 1 |
Herscheid | 1 |
Kaarst | 1 |
Kamp-Lintfort | 1 |
Kierspe | 1 |
Kirchlengern | 1 |
Lüdenscheid | 1 |
Monheim on the Rhine | 1 |
Neuenrade | 1 |
Nordkirchen | 1 |
Olfen | 1 |
Radevormwald | 1 |
Ratingen | 1 |
Rietberg | 1 |
Sendenhorst | 1 |
Siegen | 1 |
Sundern | 1 |
Verl | 1 |
Viersen | 1 |
Voerde | 1 |
Welver | 1 |
Wermelskirchen | 1 |
Wesel | 1 |
Wilich | 1 |
A color-coded thematic map of NRW shows the distribution of employees who commute by car to TU Dortmund University based on their place of residence. The cities and districts of the federal state are shown, with the colors following a logarithmic scale proportional to the number of residences:
- Dark purple: regions with the highest number of residences (logarithmic value around 3).
- Green to yellow: Regions with medium to low residential density.
- Yellow: Regions with the lowest values (close to 0 on the log scale).
Exact figures are listed in the following table:
City | Number of residences |
---|---|
Dortmund | 105 |
none | 21 |
Bochum | 17 |
Witten | 16 |
Essen | 13 |
Hagen | 9 |
Castrop-Rauxel | 8 |
Herne | 6 |
Iserlohn | 5 |
Hattingen | 5 |
Schwerte | 4 |
Lünen | 4 |
Recklinghausen | 4 |
Hamm | 3 |
Waltrop | 3 |
Dates | 3 |
Gelsenkirchen | 3 |
Lüdinghausen | 2 |
Wickede | 2 |
Sprockhövel | 2 |
Gevelsberg | 2 |
Kamen | 2 |
Fröndenberg/Ruhr | 2 |
Oberhausen | 2 |
Oer-Erkenschwick | 2 |
Selm | 2 |
Unna | 2 |
Bönen | 1 |
Kirchlengern | 1 |
Mülheim an der Ruhr | 1 |
Olfen | 1 |
Marl | 1 |
Holzwickede | 1 |
Willich | 1 |
Münster | 1 |
Menden | 1 |
Werl | 1 |
Bocholt | 1 |
Kamp-Lintfort | 1 |
Anröchte | 1 |
Weather | 1 |
Herdecke | 1 |
Neuenrade | 1 |
Dülmen | 1 |
Gladbeck | 1 |
Wuppertal | 1 |
Monheim on the Rhine | 1 |
Gütersloh | 1 |
Oberaden | 1 |
Arnsberg | 1 |
Remscheid | 1 |
Steinfurt | 1 |
A color-coded thematic map of NRW shows the distribution of students commuting by car to TU Dortmund University based on their place of residence. The cities and districts of the federal state are shown, with the coloring following a logarithmic scale proportional to the number of residences:
- Dark purple: regions with the highest number of residences (logarithmic value around 3).
- Green to yellow: Regions with medium to low residential density.
- Yellow: Regions with the lowest values (close to 0 on the log scale).
Exact figures are listed in the following table:
City | Number of residences |
---|---|
Dortmund | 63 |
Hagen | 13 |
Witten | 10 |
none | 10 |
Bochum | 9 |
Iserlohn | 9 |
Recklinghausen | 7 |
Essen | 7 |
Gelsenkirchen | 6 |
Unna | 6 |
Schwerte | 5 |
Castrop-Rauxel | 4 |
Ennepetal | 4 |
Werl | 4 |
Herne | 4 |
Fröndenberg/Ruhr | 4 |
Weather | 3 |
Schwelm | 3 |
Wuppertal | 3 |
Menden | 3 |
Waltrop | 3 |
Altena | 3 |
Lünen | 2 |
Wickede | 2 |
Werne | 2 |
Herdecke | 2 |
Oer-Erkenschwick | 2 |
Marl | 2 |
Dülmen | 2 |
Hemer | 2 |
Herten | 2 |
Cologne | 2 |
Mülheim an der Ruhr | 2 |
Arnsberg | 2 |
Ense | 2 |
Kamen | 2 |
Sprockhövel | 2 |
Gladbeck | 2 |
Sundern | 1 |
Hennef | 1 |
Gevelsberg | 1 |
Haltern am See | 1 |
Siegen | 1 |
Sendenhorst | 1 |
Lippetal | 1 |
Selm | 1 |
Halver | 1 |
Krefeld | 1 |
Oberhausen | 1 |
Lüdenscheid | 1 |
Rietberg | 1 |
Moers | 1 |
Radevormwald | 1 |
Bönen | 1 |
Ennigerloh | 1 |
Verl | 1 |
Coesfeld | 1 |
Steinfurt | 1 |
Erwitte | 1 |
Wermelskirchen | 1 |
Nordkirchen | 1 |
Herscheid | 1 |
Soest | 1 |
Wesel | 1 |
Heiligenhaus | 1 |
Dates | 1 |
Bottrop | 1 |
Voerde | 1 |
A color-coded thematic map of NRW shows the distribution of non-car commuters based on their place of residence. The cities and districts of the federal state are shown, with the coloring following a logarithmic scale proportional to the number of residences:
- Dark purple: regions with the highest number of residences (logarithmic value around 3).
- Green to yellow: Regions with medium to low residential density.
- Yellow: Regions with the lowest values (close to 0 on the log scale).
Exact figures are listed in the following table:
City | Number of residences |
---|---|
Dortmund | 557 |
Bochum | 67 |
Essen | 25 |
Witten | 11 |
Kamen | 11 |
Hamm | 11 |
Hagen | 10 |
Herne | 9 |
Duisburg | 8 |
Lunen | 8 |
Mülheim an der Ruhr | 7 |
Münster | 7 |
Unna | 5 |
Recklinghausen | 5 |
Werne | 5 |
Cologne | 4 |
Soest | 4 |
Gelsenkirchen | 4 |
Wuppertal | 4 |
Schwerte | 4 |
Ahlen | 3 |
Castrop-Rauxel | 3 |
Lüdinghausen | 3 |
Hemer | 2 |
Beckum | 2 |
Wickede | 2 |
Arnsberg | 2 |
Iserlohn | 2 |
Bielefeld | 2 |
Waltrop | 2 |
Bottrop | 2 |
Selm | 2 |
Ascheberg | 2 |
Ense | 2 |
Kierspe | 1 |
Kaarst | 1 |
Bonn | 1 |
Fröndenberg/Ruhr | 1 |
Gütersloh | 1 |
Bergkamen | 1 |
Hattingen | 1 |
Steinfurt | 1 |
Ennepetal | 1 |
Ratingen | 1 |
Oberhausen | 1 |
Heiden | 1 |
Remscheid | 1 |
Bönen | 1 |
Halver | 1 |
Dinslaken | 1 |
Menden | 1 |
Dülmen | 1 |
Holzwickede | 1 |
Welver | 1 |
Herdecke | 1 |
Marl | 1 |
Herten | 1 |
Viersen | 1 |
Balve | 1 |
Heinsberg | 1 |
A color-coded thematic map of NRW shows the distribution of car-only commuters based on their place of residence. The cities and districts of the federal state are shown, with the coloring following a logarithmic scale proportional to the number of residences:
- Dark purple: regions with the highest number of residences (logarithmic value around 3).
- Green to yellow: Regions with medium to low residential density.
- Yellow: Regions with the lowest values (close to 0 on the log scale).
Exact figures are listed in the following table:
City | Number of residences |
---|---|
Dortmund | 168 |
Bochum | 26 |
Witten | 26 |
Hagen | 22 |
Essen | 20 |
Iserlohn | 14 |
Castrop-Rauxel | 12 |
Recklinghausen | 11 |
Herne | 10 |
Gelsenkirchen | 9 |
Schwerte | 9 |
Unna | 8 |
Waltrop | 6 |
Lünen | 6 |
Fröndenberg/Ruhr | 6 |
Werl | 5 |
Hattingen | 5 |
Wuppertal | 4 |
Sprockhövel | 4 |
Ennepetal | 4 |
Oer-Erkenschwick | 4 |
Wickede | 4 |
Weather | 4 |
Kamen | 4 |
Menden | 4 |
Dates | 4 |
Hamm | 3 |
Herdecke | 3 |
Mülheim an der Ruhr | 3 |
Marl | 3 |
Arnsberg | 3 |
Schwelm | 3 |
Dülmen | 3 |
Selm | 3 |
Gladbeck | 3 |
Altena | 3 |
Gevelsberg | 3 |
Oberhausen | 3 |
Lüdinghausen | 2 |
Herten | 2 |
Bönen | 2 |
Hemer | 2 |
Werne | 2 |
Steinfurt | 2 |
Ense | 2 |
Cologne | 2 |
Haltern am See | 1 |
Hennef | 1 |
Siegen | 1 |
Bocholt | 1 |
Kamp-Lintfort | 1 |
Münster | 1 |
Holzwickede | 1 |
Willich | 1 |
Sundern | 1 |
Ennigerloh | 1 |
Halver | 1 |
Krefeld | 1 |
Olfen | 1 |
Lüdenscheid | 1 |
Rietberg | 1 |
Kirchlengern | 1 |
Heiligenhaus | 1 |
Verl | 1 |
Anröchte | 1 |
Herscheid | 1 |
Nordkirchen | 1 |
Radevormwald | 1 |
Sendenhorst | 1 |
Moers | 1 |
Monheim on the Rhine | 1 |
Remscheid | 1 |
Bottrop | 1 |
Neuenrade | 1 |
Soest | 1 |
Wermelskirchen | 1 |
Oberaden | 1 |
Gütersloh | 1 |
Erwitte | 1 |
Wesel | 1 |
Coesfeld | 1 |
Lippetal | 1 |
Voerde | 1 |
A color-coded thematic map of NRW shows the preferred commuting behavior of students and employees based on their place of residence. The cities and districts of the federal state are shown, with the colors following a logarithmic scale proportional to the preferred commuting method:
- Dark purple: regions more likely to be car commuters.
- Green to yellow: regions where car and non-car commuters are balanced.
- Yellow: Regions that are more likely to be non-car commuters.
Exact figures are listed in the following table:
(Figures of car-only and non-car are in %)
City | Car-only[%] | Non-car [%] | Residences |
---|---|---|---|
Food | 42 | 52 | 48 |
Mülheim an der Ruhr | 30 | 70 | 10 |
Oberhausen | 43 | 14 | 7 |
Remscheid | 50 | 50 | 2 |
Wuppertal | 44 | 44 | 9 |
Cologne | 33 | 67 | 6 |
Bottrop | 33 | 67 | 3 |
Gelsenkirchen | 69 | 31 | 13 |
Münster | 12 | 88 | 8 |
Dülmen | 75 | 25 | 4 |
Lüdinghausen | 40 | 60 | 5 |
Castrop-Rauxel | 80 | 20 | 15 |
Herten | 67 | 33 | 3 |
Marl | 75 | 25 | 4 |
Recklinghausen | 65 | 29 | 17 |
Waltrop | 67 | 22 | 9 |
Steinfurt | 67 | 33 | 3 |
Gütersloh | 50 | 50 | 2 |
Bochum | 27 | 68 | 98 |
Dortmund | 21 | 69 | 802 |
Hagen | 67 | 30 | 33 |
Hamm | 18 | 65 | 17 |
Herne | 50 | 45 | 20 |
Ennepetal | 80 | 20 | 5 |
Hattingen | 71 | 14 | 7 |
Herdecke | 75 | 25 | 4 |
Witten | 65 | 28 | 40 |
Arnsberg | 50 | 33 | 6 |
Halver | 50 | 50 | 2 |
Hemer | 50 | 50 | 4 |
Iserlohn | 88 | 12 | 16 |
Menden | 67 | 17 | 6 |
Ense | 50 | 50 | 4 |
Soest | 20 | 80 | 5 |
Wickede | 67 | 33 | 6 |
Bönen | 67 | 33 | 3 |
Fröndenberg/Ruhr | 75 | 12 | 8 |
Holzwickede | 50 | 50 | 2 |
Kamen | 22 | 61 | 18 |
Lunen | 40 | 53 | 15 |
Schwerte | 64 | 29 | 14 |
Selm | 50 | 33 | 6 |
Unna | 53 | 33 | 15 |
Werne | 29 | 71 | 7 |
Duisburg | 0 | 80 | 10 |
Ratingen | 0 | 100 | 1 |
Grevenbroich | 0 | 0 | 1 |
Kaarst | 0 | 100 | 1 |
Viersen | 0 | 100 | 1 |
Dinslaken | 0 | 100 | 1 |
Bonn | 0 | 100 | 1 |
Heinsberg | 0 | 100 | 1 |
Heiden | 0 | 100 | 1 |
Ascheberg | 0 | 100 | 2 |
Ahlen | 0 | 100 | 3 |
Beckum | 0 | 100 | 2 |
Bielefeld | 0 | 100 | 2 |
Balve | 0 | 100 | 1 |
Kierspe | 0 | 100 | 1 |
Welver | 0 | 100 | 1 |
Bergkamen | 0 | 100 | 1 |
Heiligenhaus | 100 | 0 | 1 |
Monheim on the Rhine | 100 | 0 | 1 |
Grevenbroich | 0 | 0 | 1 |
Willich | 100 | 0 | 1 |
Kamp-Lintfort | 100 | 0 | 1 |
Moers | 50 | 0 | 2 |
Voerde | 100 | 0 | 1 |
Wesel | 100 | 0 | 1 |
Radevormwald | 100 | 0 | 1 |
Wermelskirchen | 100 | 0 | 1 |
Hennef | 100 | 0 | 1 |
Bocholt | 100 | 0 | 1 |
Coesfeld | 100 | 0 | 1 |
Nordkirchen | 100 | 0 | 1 |
Olfen | 100 | 0 | 1 |
Dates | 100 | 0 | 4 |
Gladbeck | 100 | 0 | 3 |
Haltern am See | 100 | 0 | 1 |
Oer-Erkenschwick | 100 | 0 | 4 |
Ennigerloh | 100 | 0 | 1 |
Sendenhorst | 100 | 0 | 1 |
Rietberg | 100 | 0 | 1 |
Verl | 100 | 0 | 1 |
Kirchlengern | 100 | 0 | 1 |
Gevelsberg | 100 | 0 | 3 |
Schwelm | 100 | 0 | 3 |
Sprockhövel | 100 | 0 | 4 |
Weather | 80 | 0 | 5 |
Sundern | 100 | 0 | 1 |
Altena | 100 | 0 | 3 |
Herscheid | 100 | 0 | 1 |
Lüdenscheid | 100 | 0 | 1 |
Neuenrade | 100 | 0 | 1 |
Siegen | 100 | 0 | 1 |
Anröchte | 100 | 0 | 1 |
Erwitte | 100 | 0 | 1 |
Lippetal | 100 | 0 | 1 |
Werl | 100 | 0 | 5 |
The figure above shows that the city of Dortmund forms the center of TU Dortmund University's catchment area. The data also shows that many commuters also come from surrounding cities and municipalities, particularly along the Ruhr axis. On average, both employees and students travel 19 km (median: 10 km) per commute. Students (employees) need an average of 39 (33) minutes to cover this distance. While the commuting frequency for employees hardly differs between the lecture period with 3.8 (median: 4.0) days per week and the lecture-free period with 3.6 (median: 4.0) days per week, students are at the university on average 3.7 (median: 4.0) days per week during the lecture period and 2 (median: 2) days per week during the lecture-free period.
The survey showed that people tend to use their car instead of public transport or cycling, especially in residential areas north and south of Dortmund. There is less car use along the east-west axis. A more detailed breakdown can be seen in the adjacent figure.
The survey also shows that a significant proportion of people living in Dortmund also travel to the university by car. Despite the good public transport connections and possible cycle paths, around 21% of respondents use the car as their primary means of transportation.
Average Distances and Typical Commuting Routes
Average distances can be calculated from the survey depending on the type of mobility. These can be seen in the adjacent graph. It is striking that the shortest distances are covered by bicycle/pedestrian transport (4.2 km), followed by local public transport (19.4 km) and private motorized transport (26.5 km). The longest distances are covered by long-distance rail passenger transport (SPFV, 41.6 km).
If a typical, average commute for students (TU employees) is calculated on the basis of the survey using the actual kilometers driven, then 7.4 (10.8) km would be driven by car in the morning, 0.7 (0.7) km would be covered after changing to public transport, and then 9.5 (5.3) km would be covered by public transport. The last part of the journey of 1.1 (2.0) km would be covered by bicycle or on foot. This is clearly illustrated in the diagram above.
Mobility Behavior
Interactive graphic. Source: Sustainability Office/TU Dortmund University
Of all the people who took part in the survey, 38% use a car or private car as their primary means of transportation. However, a breakdown by status group reveals differences: 49% of employees travel by car, while the proportion of students is around 30%.
The picture is reversed for public transport. Here, the proportion of employees is around 30%, while just under 67% of students use public transport. 52% of employees and 45% of students travel by bicycle/foot.
It is striking that employees tend to use private motorized transport more often for their daily commute, while students mostly choose public transport. Nevertheless, around a third of them also regularly use the car to get to the university.
The stacked bar chart shows the distance travelled per mobility type (normalized to the average distance) for the status groups (non-)scientific employee and students.
The individual mobility types are color-coded:
- Yellow: bicycle/ on foot
- Green: Public transport (local public transport)
- Blue: Train, long-distance transport
- Dark blue: Motorized private transport (car, motorcycle, etc.)
Exact figures can be found in the following table:
(in km)
(Non-) scientific employee | Student | |
---|---|---|
Bicycle / on foot | 1,97 | 1,08 |
Public transport | 5,28 | 9,51 |
Train / long-distance traffic | 0,67 | 0,74 |
Motorized private transport | 10,82 | 7,42 |
The average number of modes of transport used on the way to university is 1.6, with a median of 1.0, meaning that most participants mainly use one mode of transport, in contrast to the hypothetical commute shown above, where four modes of transport were used.
Carpooling
It is not possible to evaluate this question as it was asked too unspecifically. It is unclear whether the question refers to the people in the vehicle or to the number of additional people in the vehicle.
Of the 519 people who came to the university by car, 52 people (10 %) stated that they were traveling in a carpool. These figures suggest that the proportion of people who carpool to TU Dortmund University may be higher at TU Dortmund University than the national average: according to the Federal Environment Agency, the Federal Ministry of Transport's "Mobility in Germany 2008" report shows that around five percent of car journeys to work are made as passengers and around 65 percent of journeys to work are made as drivers. In comparison, TU Dortmund University appears to have a carpooling rate that is twice as high.
The TU in Comparison
The figures collected in the survey are in line with the results of other mobility surveys. A comparison can be made with the InnaMo Ruhr survey from 2021. In this survey, members of the University Alliance Ruhr (UA-Ruhr, consisting of Ruhr-Universität Bochum, TU Dortmund University and the University of Duisburg-Essen) were asked about their mobility behavior. As Ruhr-Universität Bochum and the University of Duisburg-Essen are very similar to TU Dortmund University, both are also located in the Ruhr region, are similar in size and connected to a highway, offer similar capacities for motorized private transport and are similarly easily accessible by public transport, they are well suited for a comparison.
The survey showed that on average 31% of university members use their car to get to university before the coronavirus lockdown and 39% during the lockdown. This is in line with the 38% from this survey. The InnaMo Ruhr survey also shows that 49% of people traveled to the university by public transport before the lockdown. This also matches the results of this survey, in which 67% of all students and 30% of all employees used public transport.
Another mobility survey conducted by the Frankfurt University of Applied Sciences (UAS) shows similar ratios to the present survey, but differences in location should be taken into account, as the UAS is significantly smaller (in terms of area, number of students) than the UA-Ruhr universities and is located fairly centrally in Frankfurt. Despite the central location of UAS, 38% of all university members come to the university by car and 36% by public transport.
Other mobility surveys from the RheinMain University of Applied Sciences or the Bochum University of Applied Sciences show some clear differences in the use of the individual modality types, although similar trends in the use of modality types between employees and students are also evident here.